
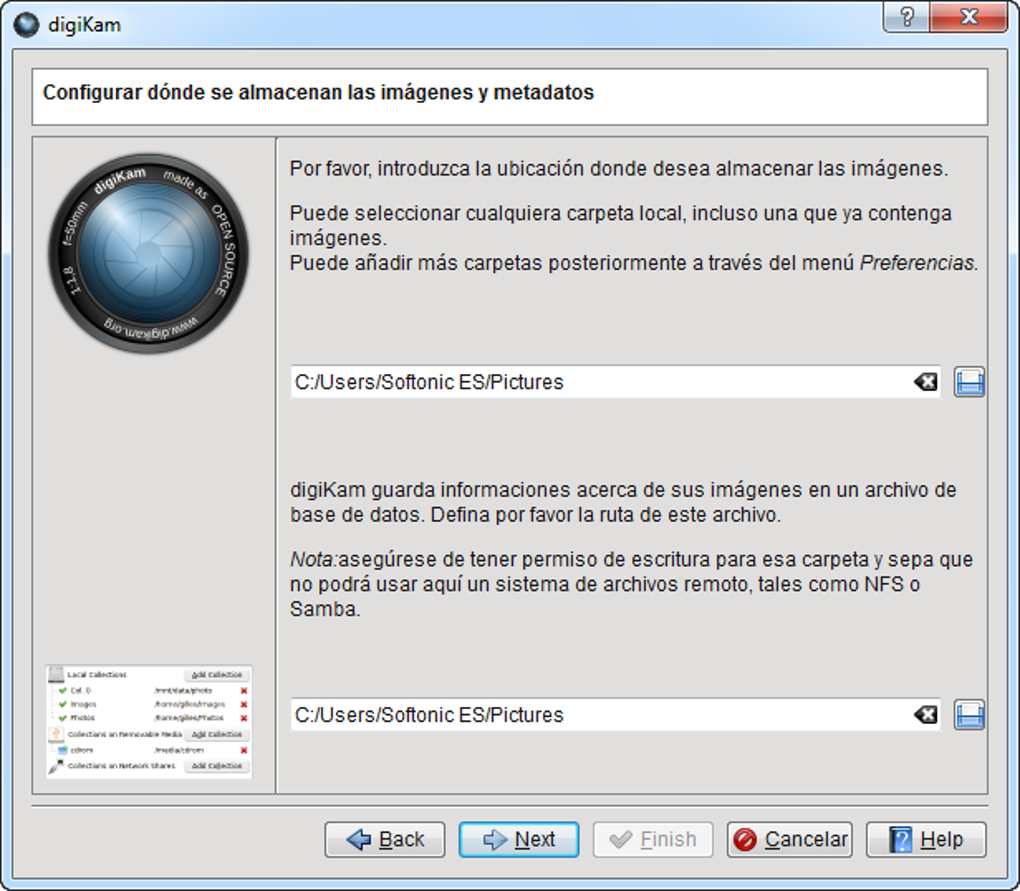
We have saved coding time, run-time speed, and a improved the success rate which reaches 97% of true positives. No learning stage is required to perform face detection and recognition. The new code, based on recent Deep Neural Network features from the OpenCV library, uses neuronal networks with pre-learned data models dedicated for the Face Management. The goal of this project was to leave behind all the old ideas and port the detection and the recognition engines to more modern deep-learning approaches.
DIGIKAM REVIEW 2019 CODE
We tried again this year, and a complete rewrite of the code was successfully completed by a new student named Thanh Trung Dinh. The approach to resolve the problem took a wrong turn and that is why the deep learning option in Face Management was never activated for users. We saw this as a technical proof of concept, but not usable in production. The result was mostly demonstrative and very experimental, with poor computation speed results. Also, with the user feedback from bugzilla, Face Recognition does not provides a good experience when it comes to an auto-tag mechanism for people.ĭuring the summer of 2017, we mentored a student, Yingjie Liu, who worked on the integration of Neural Networks into the Face Management pipeline based on the Dlib library. Face detection is able to give 80% of good results, while analysis is not too bad, but requires a lot of user feedback to confirm whether or not what it has detected is really a face. This does work, but does not provide a high level of positive results. Up until now, the complex methodologies that analyzed image contents to isolate and tag people’s faces were performed using the classical feature-based Cascade Classifier from the OpenCV library. It had the peoblem of not being powerful enough to facilitate the faces-management workflow automatically. The algorithms used in the background (not based on deep learning) were old and had been unchanged since the first revision that included this feature (digiKam 2.0.0). De complete release notes voor deze uitgave zien er als volgt uit: Deep-Learning Powered Faces Managementįor many years, digiKam has provided an important feature dedicated to detecting and recognizing faces in photos. In versie 7.0 is met name de gezichtsherkenning sterk verbeterd. Meer over de mogelijkheden van dit programma is op deze pagina te vinden. Het bevat ondersteuning voor uiteenlopende bestandsformaten en kan daarnaast via plug-ins van meer mogelijkheden worden voorzien, zoals het exporteren van een album naar Facebook of Flickr. Deze fotobeheer- en beeldbewerkingsapplicatie heeft downloads voor Linux, BSD, macOS en Windows.
